Seeing the Earth for the Forests
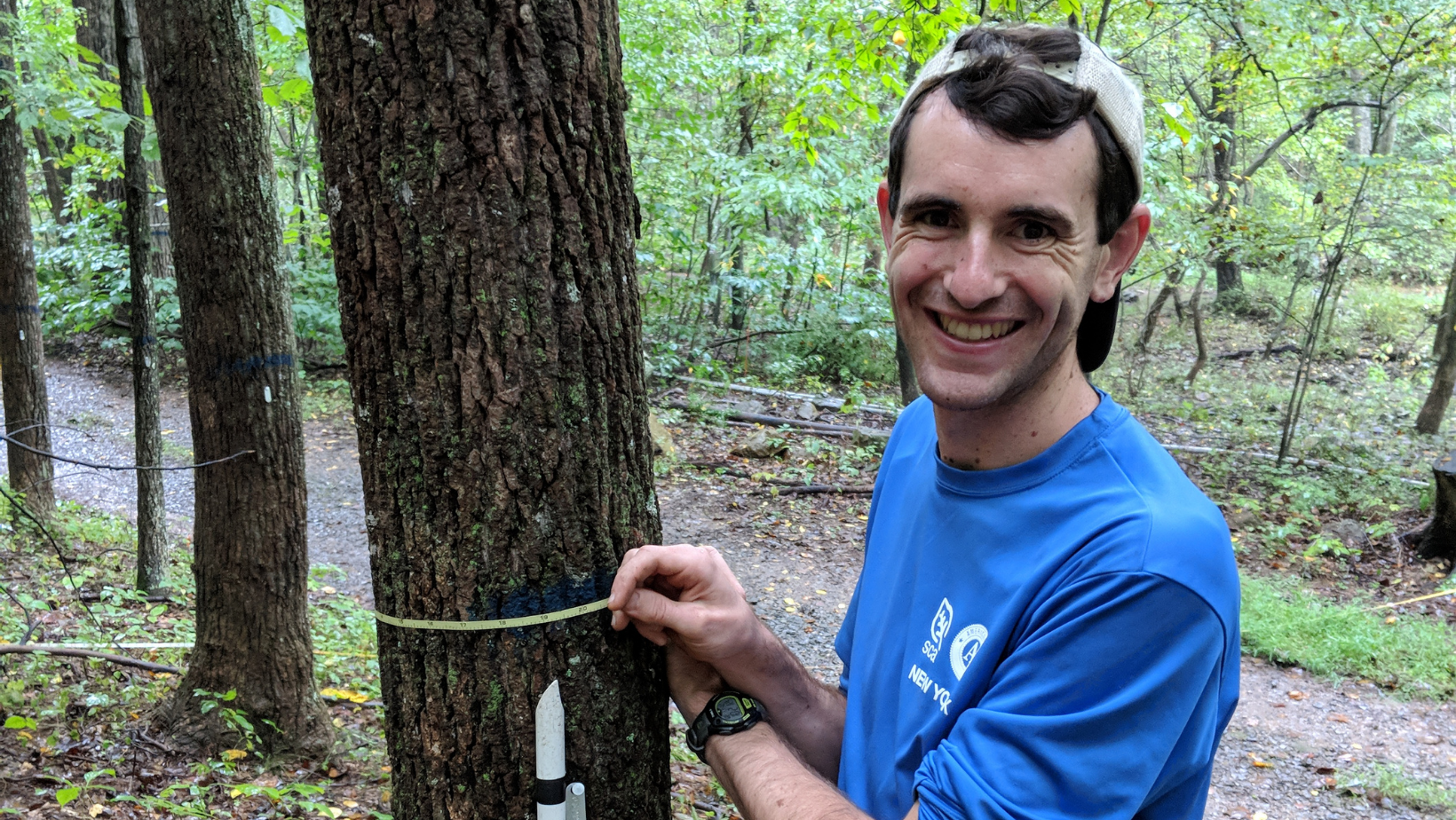
Guest post: Ian McGregor is studying geospatial analytics at North Carolina State University. He was selected as an NC Space Grant 2020-21 Graduate Research Fellow. Soon after, he accepted an award from the NASA FINESST program to expand his research on using satellite data to analyze changes in forests. Read about McGregor and other fellows in our previous post.
Origins
Coming from southern California, I had a very West-Coast idea of what forests were. They were Ponderosas interspersed with Jeffrey pines and Douglas-firs, or stands of Pacific madrone, California bay-laurel, and redwoods up north. But tropical forests or forests that lost their leaves and changed color? That was something I only saw on film or in pictures from National Geographic.
When I finally saw one, you could say it was love at first sight. The beaches of my hometown will never leave me, but, wow, was I amazed to learn about and work in deciduous hardwood forests – these were real maples! Balsam fir leaves in my hand, birch trees all around! I enjoyed all of it, and ever since that first experience building trails and backpacking with the Student Conservation Association in New York’s Adirondack State Park, I’ve never looked back. Since then, I’ve gone on to analyze fire regimes in Gabonese tropical forests for my Master’s of Science degree at University of Oxford and then coordinate dendrochronological research during an internship with the Smithsonian Conservation Biology Institute.
Recently I decided on a new approach to look at forests from the sky – literally – using satellite imagery. I initially intended to use my experience with the Smithsonian (northern Virginia) to research the Southeast, especially with regard to fire and invasive pests. I was lucky enough to be funded by NC Space Grant; then soon after, the NASA FINESST program allowed me the chance to broaden my research scope to a larger case-study in another southeast region. Now, I’m analyzing forest disturbances in southeast Asia, specifically, Myanmar.
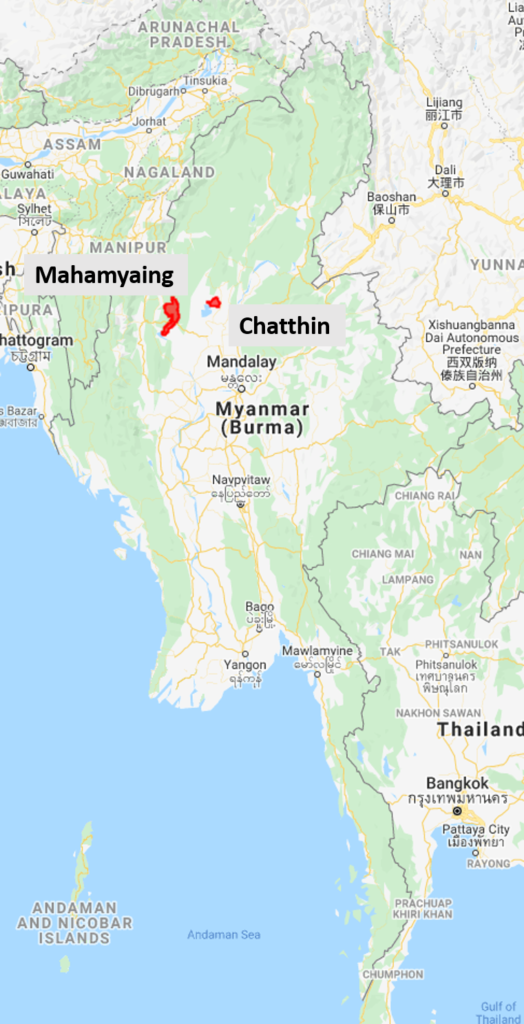
Research Background
“Myanmar is one of the most-forested countries in Southeast Asia.”
If you read any scientific paper regarding deforestation in the country, I can almost guarantee you a version of this sentence will be in the introduction, for good reason. A few decades ago, Myanmar had upwards of 60% forest cover, which made it a stronghold for endangered species like the Eld’s deer. Yet with the democratization of the country, deforestation — primarily at the hands of agribusiness — has become rampant. Some areas like the Ayeyarwady Delta region, for example, are projected to have lost all non-protected mangrove forest within the next 10 years.
Like other countries, Myanmar has its own version of national parks. However, many are not able to efficiently protect the flora and fauna within park boundaries due to a lack of resources, be it financial or personnel. Chatthin Wildlife Sanctuary is one of the exceptions, and the Smithsonian has partnered with park staff there since the 1990s. Located in north-central Myanmar and containing one of the last remaining landscapes of tropical dry (deciduous) forests, the park is one of the last strongholds for the Eld’s deer. And yet, even with moderate resources, deforestation still occurs. Outright logging is illegal but monitoring can be difficult over large areas, especially when there is widespread use of forest products by locals. This is where my partnership with the Smithsonian comes into the picture. Through them, I have an avenue of engagement with the local NGO who helps with Chatthin’s conservation activities.
Current Research Activities
I reached out to connections at the Smithsonian, detailing my remote sensing skills and my desire to assist with an ongoing conservation issue. Luckily, the Chatthin park staff had expressed interest to their partner NGO to mitigate deforestation, and specifically were keen on stopping deforestation activities during the act, instead of discovering the results of them weeks later. The NGO reached out to the Smithsonian, which then suggested the project to me.
I was excited to help, and started collecting the necessary data for developing my workflow. In my mind, this project has two main objectives: first, progress technical abilities of near real-time disturbance monitoring, and second, have the resultant algorithm / code / method as user-friendly and accessible as possible such that park staff can refine and use the research to yield tangible change.
At its technical core, my research aims to improve forest disturbance monitoring by decreasing the amount of time between a section of forest being cut down and when we can detect this via satellite, also known as “reducing temporal / detection latency.” Let’s take an example:
Imagine we have a point within Chatthin for which we have an exact date of disturbance. Using long-term observations from 3 different satellite sensors, I fit a time series model to each one and then calculate the value difference between the fit model and the actual observation (this is called the model residual). These residuals — standardized — constitute their own unitless time series for each sensor, which I then combine into a single, aggregate series.
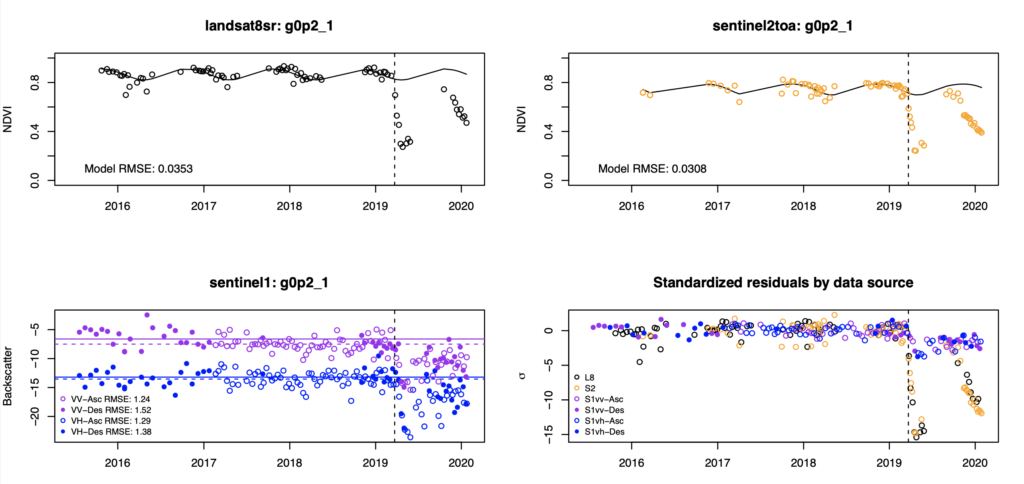
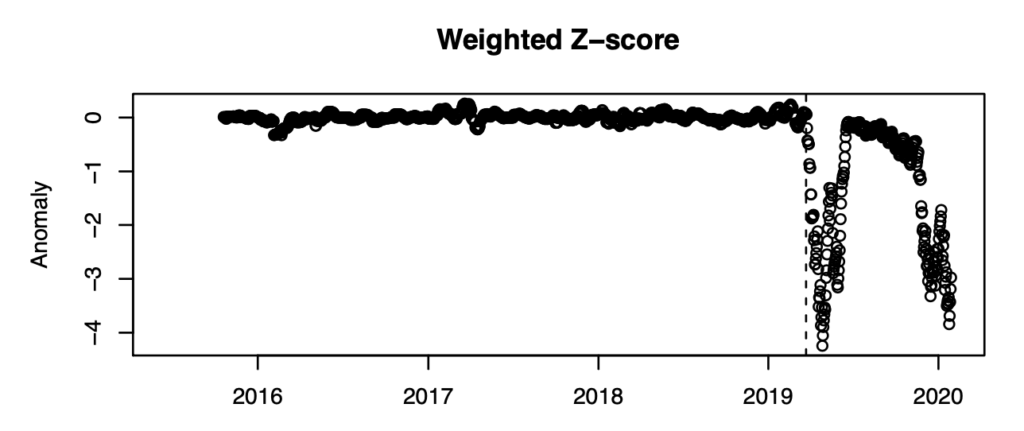
After applying a weighting function to them, I get something like this, where the disturbance signal is clear.
We then map this to a probability of disturbance, giving us the time series below. If we put a threshold probability of 75%, then we can say we confidently detect the disturbance in 3 days, with 0% false positive (disturbance didn’t happen prior to actual disturbance, but the probability was >75%), and 5.7% false negative (disturbance did happen after actual disturbance date, but the probability was < 75%).
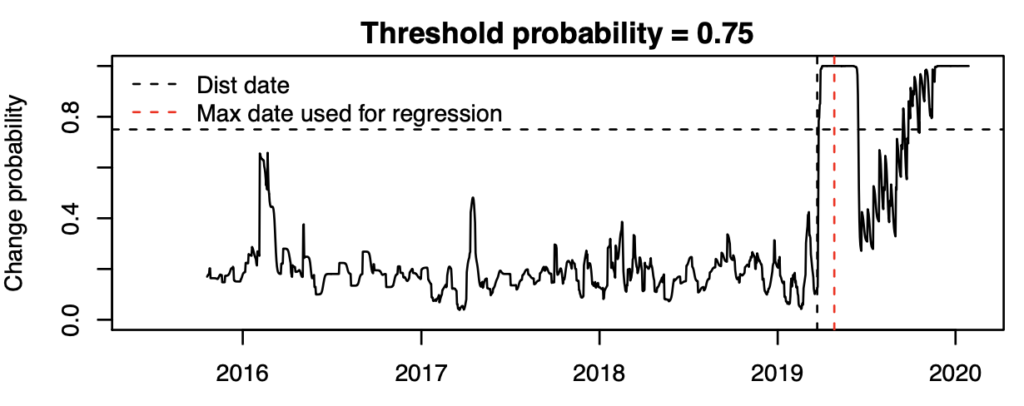
Goals and the Future
Of course, this is for only one point, and in a real-world context we want to have this be automatic and accurate over a wide area, taking into account biophysical and socioeconomic variables. To achieve this, I’ve collected training data (like this point) and I will be statistically combining prior knowledge with each day’s probability (i.e. Bayesian methodology) to perform at a landscape scale over all of Chatthin.
My hope is that, not only will this help park staff to more quickly identify forest disturbances, but eventually the method can be accessed via a Google Earth Engine app, where users can independently verify deforestation occurrences. By providing park staff decreased latency and deforestation probabilities instead of a binary either deforestation happened or it didn’t, my goal is that they will be better equipped to more effectively allocate management resources.
Having never known about remote sensing before I started my Ph.D., I am delighted by the implications of my research and the ability of it to contribute to meaningful change. As this project combines my experience in forest ecology with my recent coursework, I anticipate that after graduation I will extend this kind of application with a postdoc in ecological remote sensing. Analyzing forests from space is a long way from beaches, Ponderosas, and redwoods, but I am excited to continue supporting the conservation of those forests that I once only saw on a TV screen.
- Categories: