Mapping North Carolina’s Vulnerable Estuarine Shorelines
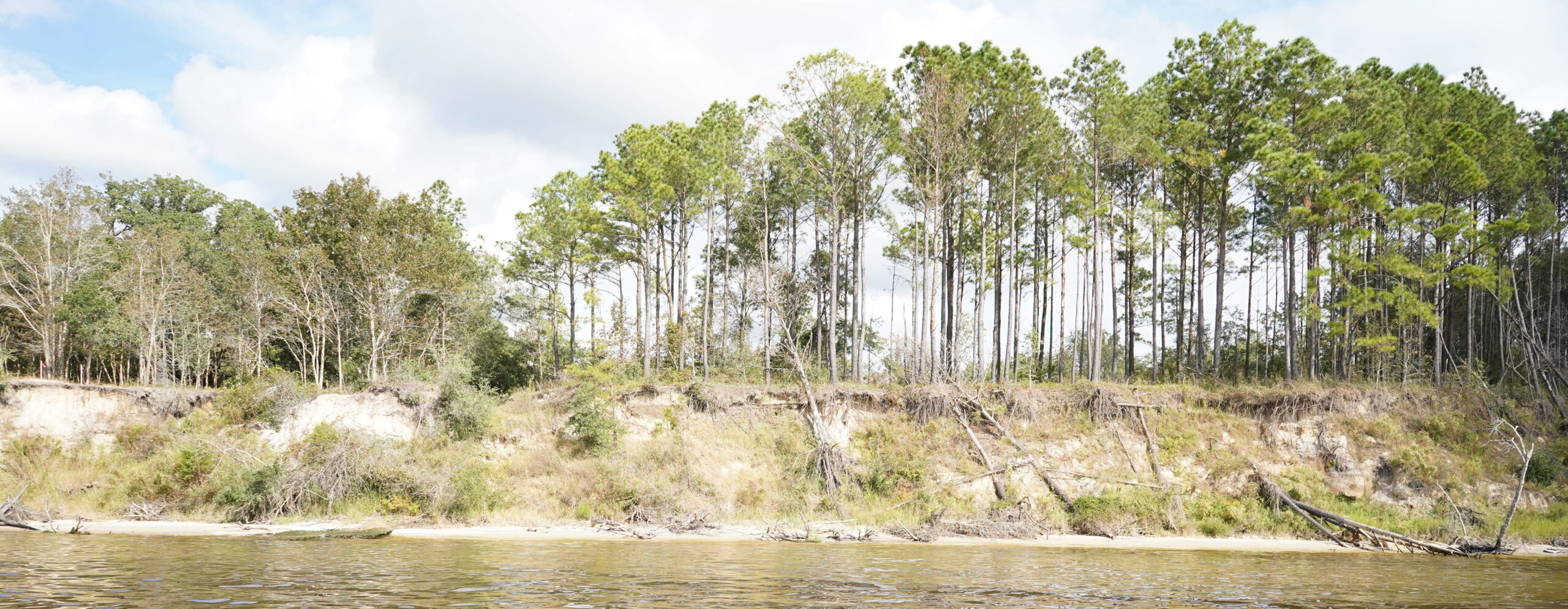
BY JESSICA RICHTER
Beginnings and Motivations
An important lesson I have learned throughout my academic career is that there is no “correct” path to achieve your goals. A diverse range of experiences have led me to find my passion in remote sensing and coastal resiliency and brought me to where I am today.
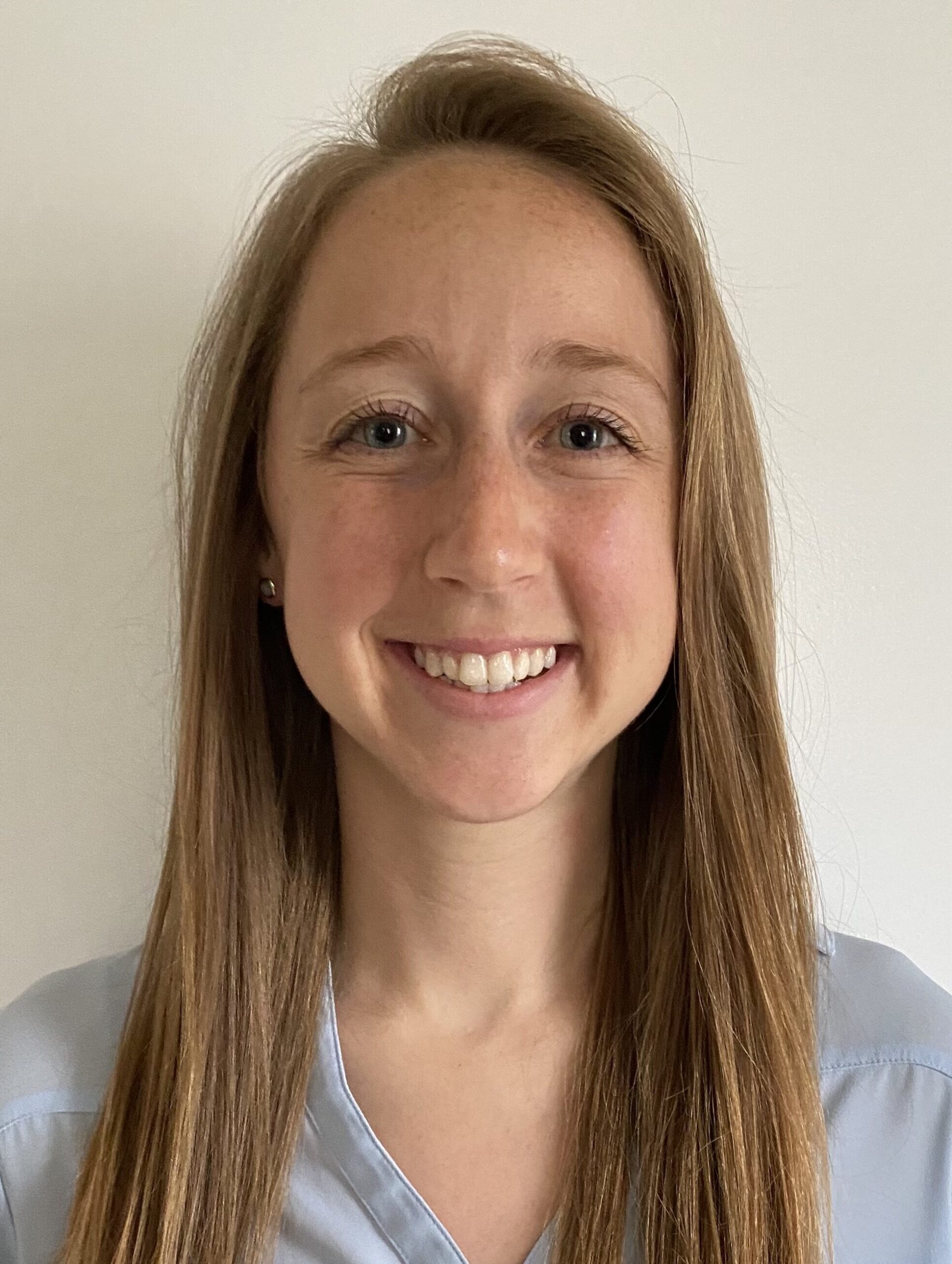
My academic career began at Eckerd College in St. Petersburg, Florida, where I pursued a bachelor’s degree in marine science and minored in computer science and mathematics. There, I was able to build on my passion for coastal and oceanographic science by learning how geospatial technologies can inform ecosystem management. Next, I spent three years in Vermont working at a regional planning commission. In this role, I conducted road erosion inventories, also known as REIs, throughout the east-central Vermont region. These inventories established baseline conditions of road segments within 100 feet of a water resource and identified how municipalities can reduce stormwater-related erosion on these segments. This led me to think about the relationship between erosion and watershed health on a larger scale. I became interested in investigating how erosion, particularly coastal erosion, changes the landscape and impacts community resiliency over time.
I decided to build upon this interest by pursuing a graduate degree at East Carolina University. In this pursuit, my aim was to conduct research that would harmonize my past experiences while expanding my knowledge of remote sensing and geospatial analysis. I connected with Dr. Hannah Sirianni, my academic advisor – and mentor for my NC Space Grant-North Carolina Sea Grant joint graduate research fellowship – and determined a research plan that would align with the mission of her Coastal Geography & Terrain Analysis Lab, as well as my overall interest in coastal erosion and resiliency. From there, my focus landed on investigating how machine learning ensembles and object-based image analysis (OBIA) can be used to classify estuarine shorelines within the Neuse River Estuary (NRE) in North Carolina.
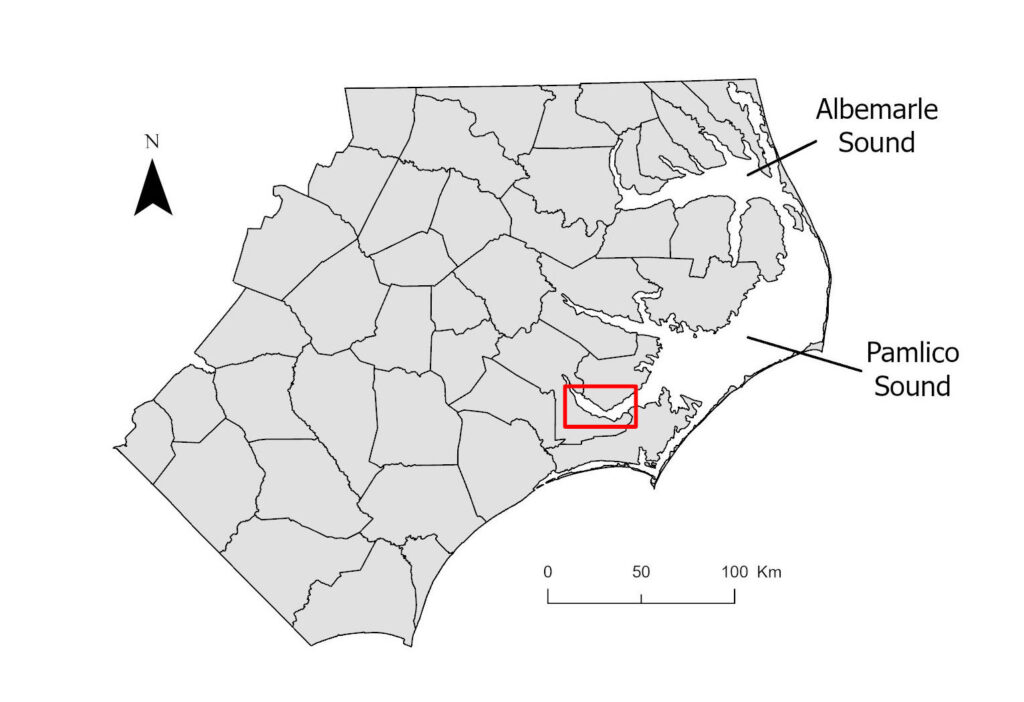
The NRE is a major tributary to the Albemarle Pamlico Estuarine System (APES), the second-largest estuarine complex in the United States. The APES and its tributaries are experiencing significant shoreline change in response to extreme storms and rising sea-levels. A significant portion of extreme storms have impacted the NRE over the past three decades. In 2018, Hurricane Florence devastated communities along the NRE, as storm-force waves eroded east-facing bluffs. The largest localized bluff retreat was above 20 meters, significantly greater than the retreat observed from past hurricanes.
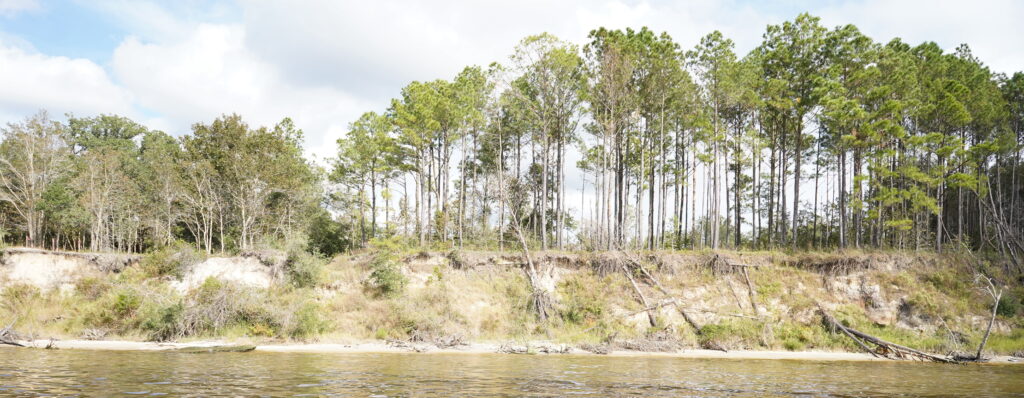
An accurate and up-to-date shoreline classification map of the NRE is critical to understanding the context and magnitude of storm-induced erosion and would enable coastal communities to better understand erosion risks associated with different shoreline environments. This is what ultimately inspired me to focus my research on assessing advanced digital image processing and classification techniques to improve shoreline maps in the NRE.
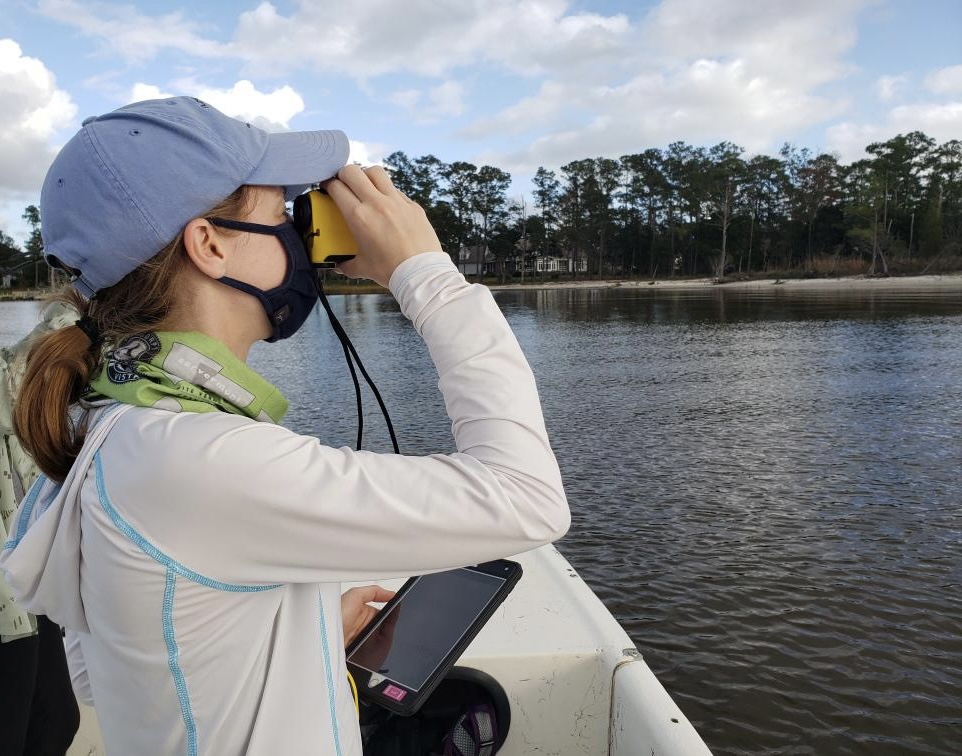
When I learned about the NC Space and Sea Grant Joint Graduate Fellowship, I believed that the interdisciplinary nature of my research aligned well with the mission and strategic plans of both organizations. I was thrilled to ultimately receive the fellowship and gain support for this research.
Skills Gained
With the support of the fellowship, I was able to refine many in-demand skills while conducting research, including streamlining remote sensing data processing and analysis. To predict shoreline classifications within the NRE, I trained five machine learning algorithms based on high-resolution aerial imagery and LiDAR elevation data. I also created scripts using Python and R, respectively, to automate aerial imagery processing and compute machine-learning ensemble results. An ensemble analysis refers to the use of multiple algorithms to make a decision and has been shown to improve classification accuracies in many environments.
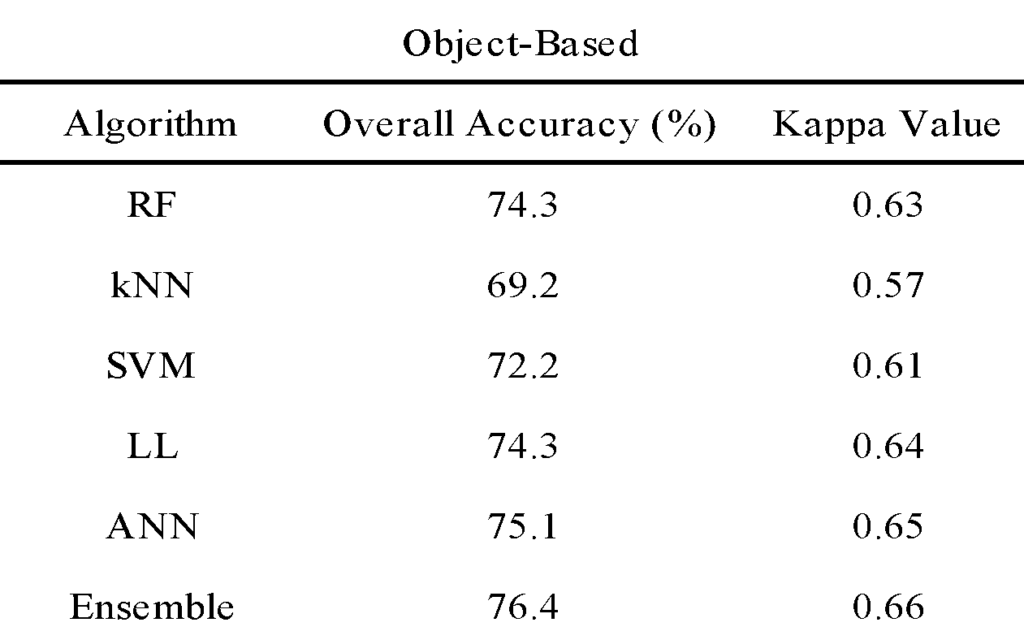
Through my research, I found that a machine learning ensemble was able to best predict estuarine shoreline classes in the NRE with an overall accuracy of 76.4%, compared to the five individual algorithms. Kappa values, in this study, are used to assess the level of agreement within a classifier and take into account the potential for agreement occurring by chance. Based on the scheme developed by Landis and Koch (1977), the ensemble results showed substantial agreement beyond chance.
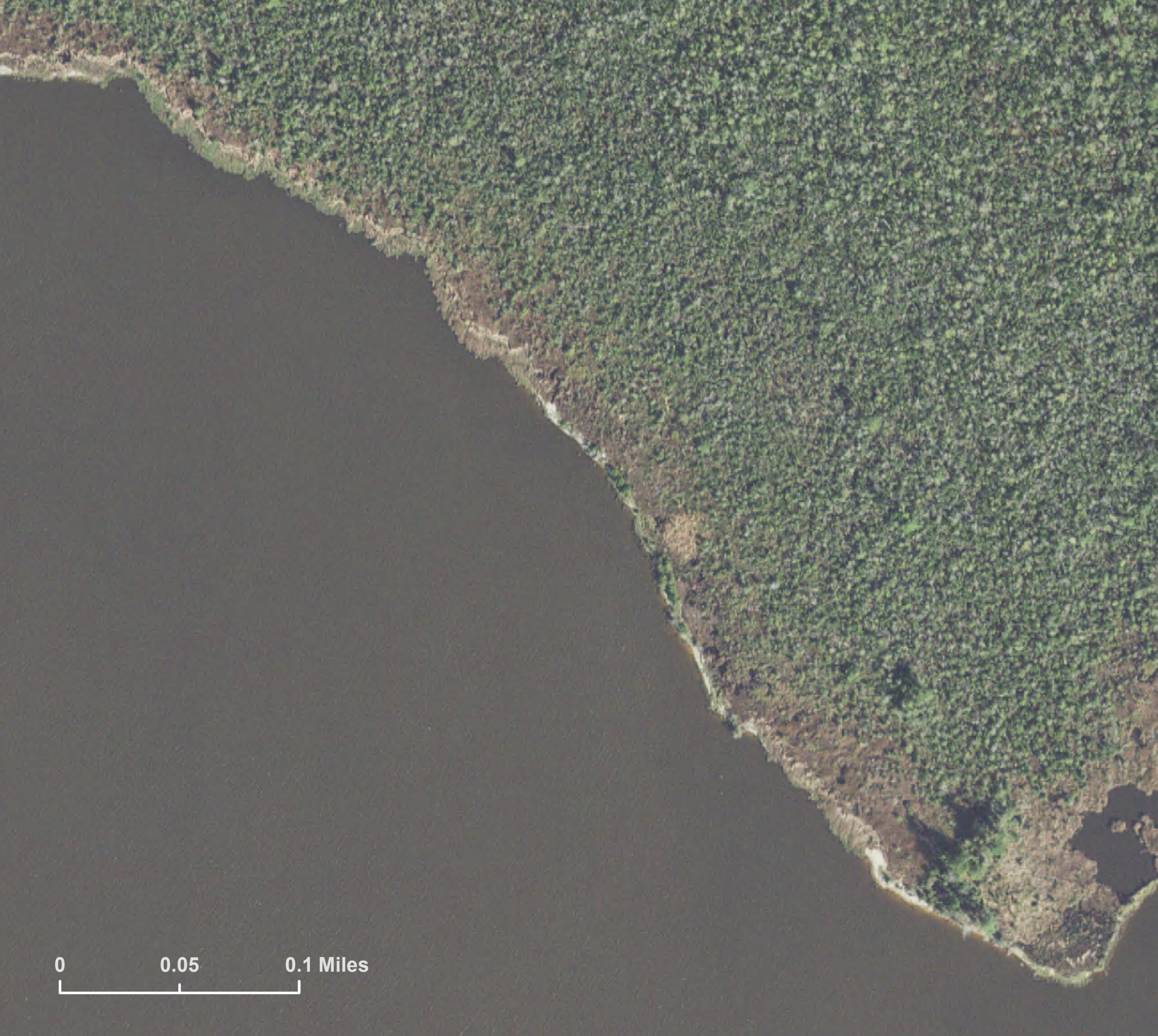
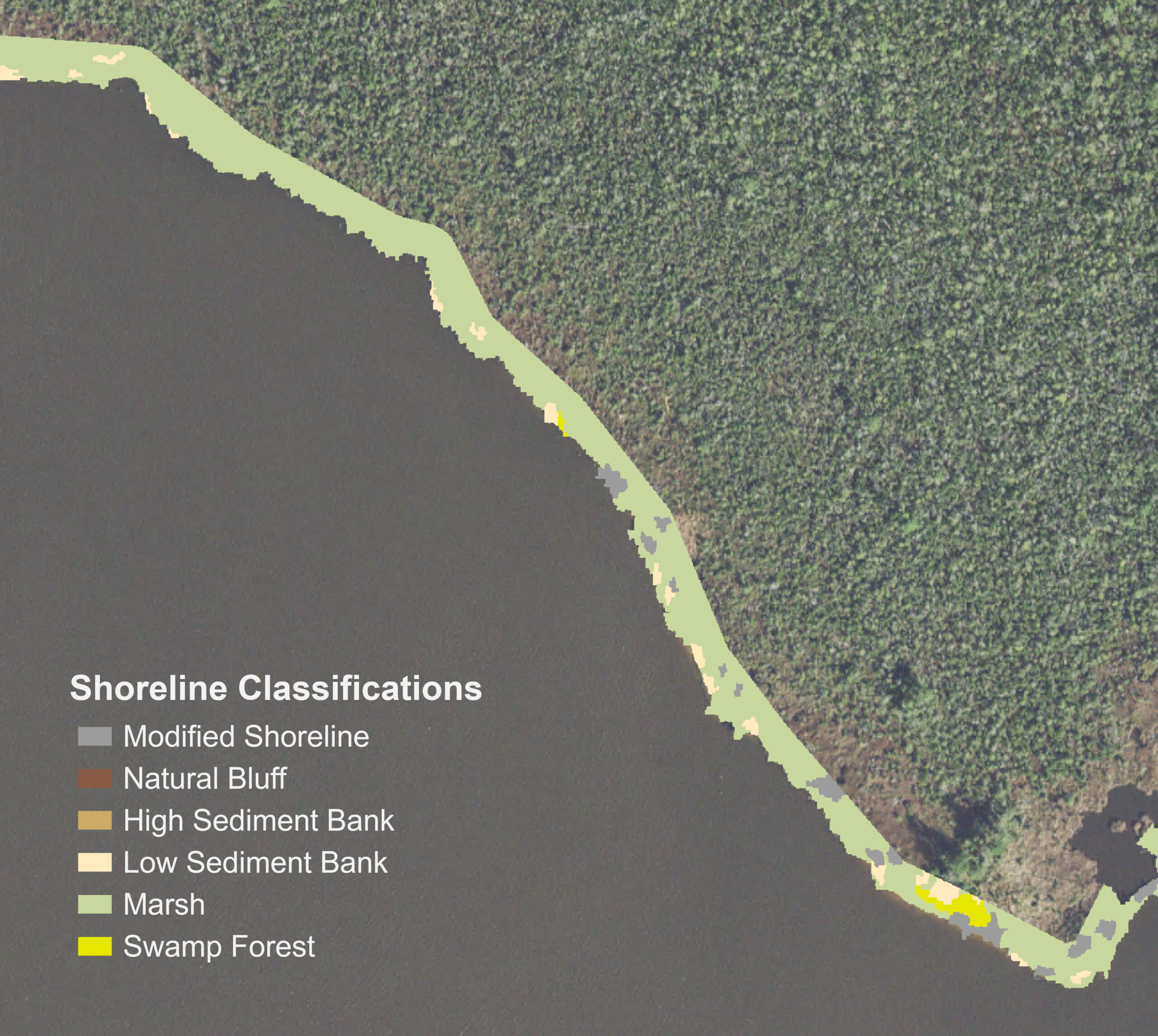
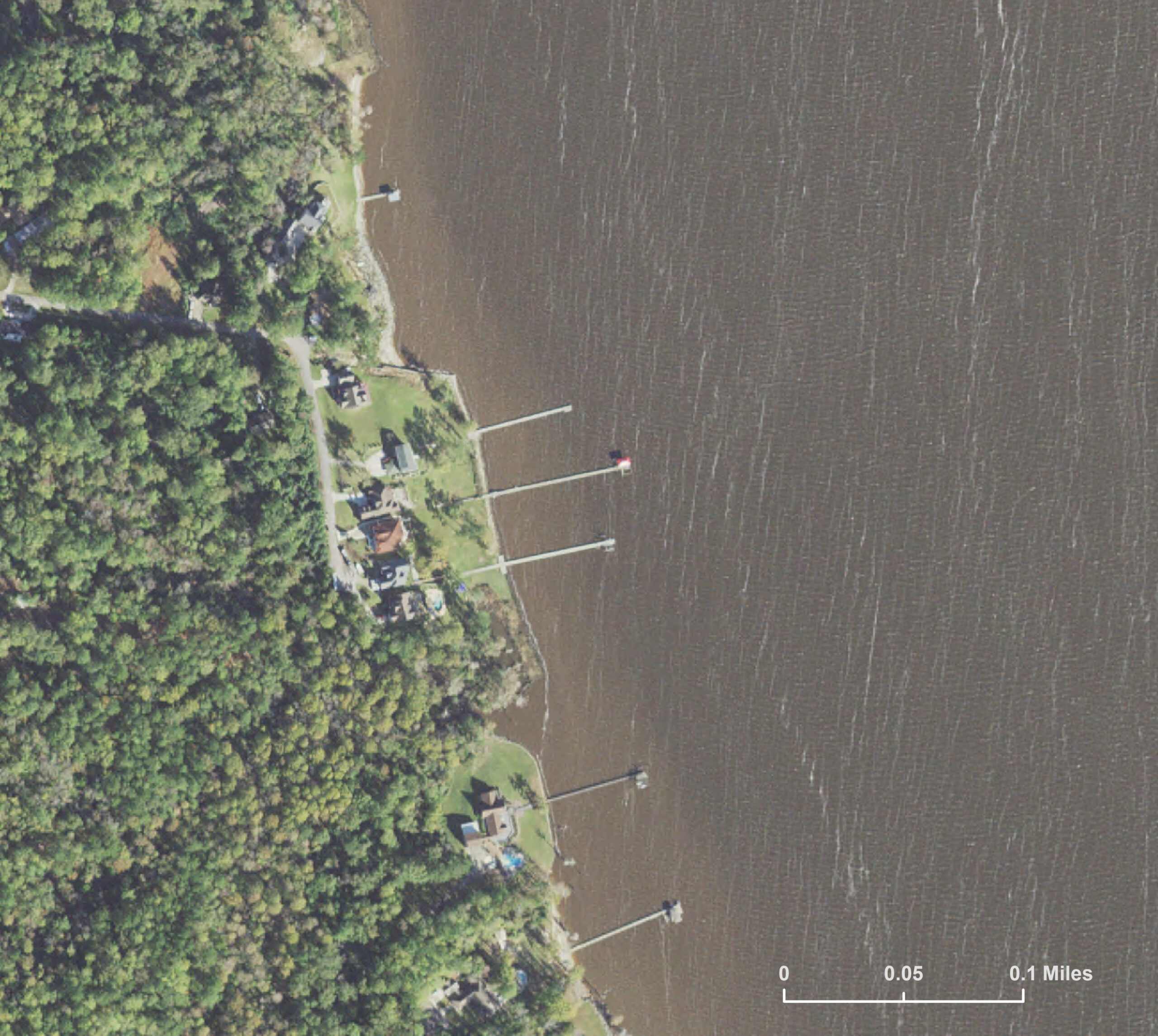
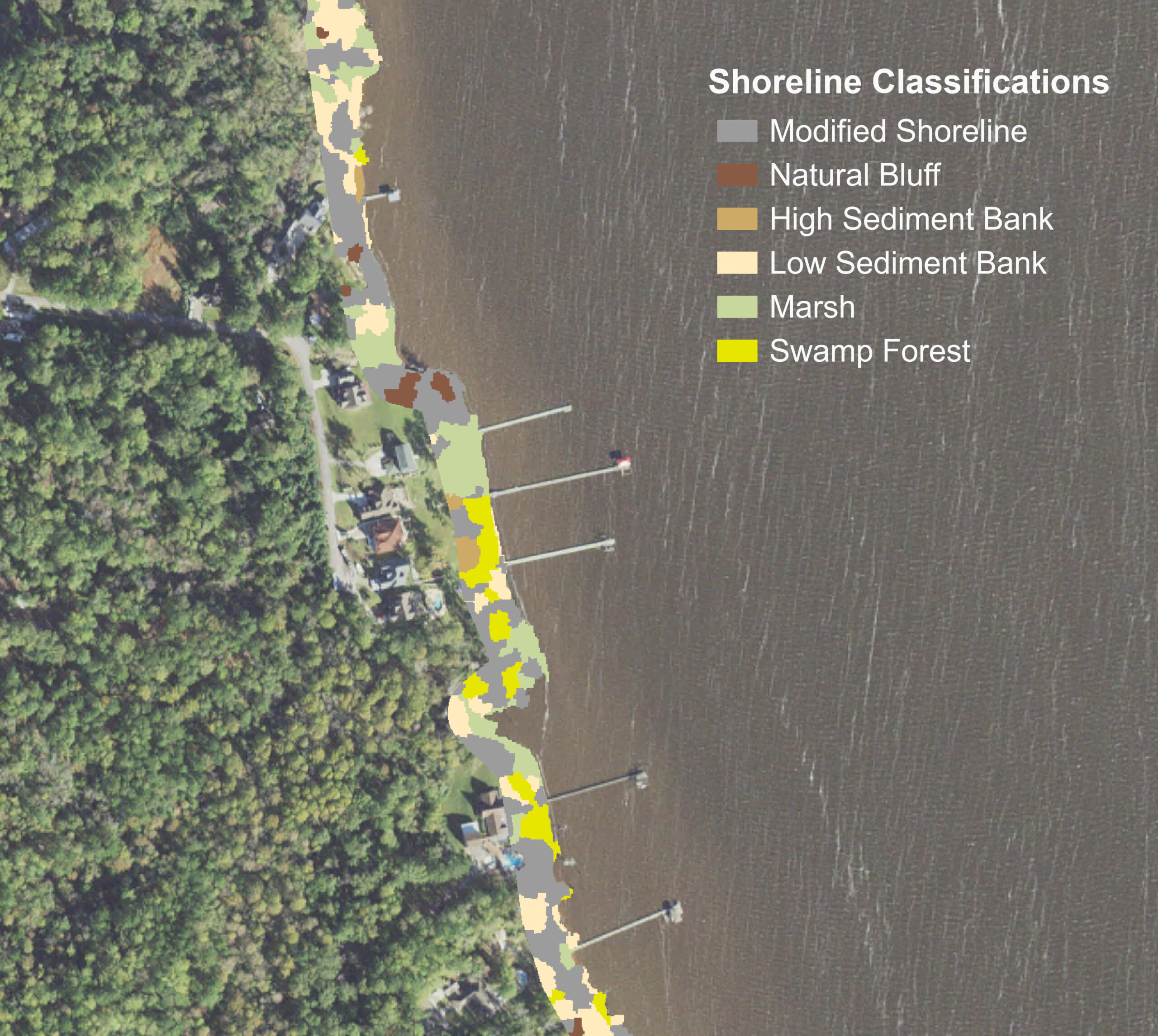
The fellowship also gave me the opportunity to improve my communication skills to both scientific and non-scientific audiences. I presented research results at the 2021 American Geophysical Union (AGU) Conference, as well as the 2022 NC Space Symposium. Both presentations took the form of a lightning talk, which pushed me to be concise and focus on the bigger picture.
To reach a broader audience, the shoreline classification maps will be available on ArcGIS Online and a StoryMap of the process will be made available as well.
Moving Forward
Next, I will be working as a GIS Research Analyst for the World Resources Institute in Washington, DC. Many of the skills I gained through this fellowship will help me succeed in this new role. They include high-level spatial analysis, satellite imagery interpretation, and effective communication to collaborate with internal and external partners. I am eager to step into this new role and apply my geospatial skills to forest management and tree cover loss.
Although a Ph.D. is not in my immediate future, there are many ways I would like to expand upon my research if I pursue this path further. Primarily, I would like to explore how deep learning, especially convolutional neural networks (CNNs), could further improve shoreline classification accuracy in estuarine environments. Machine learning approaches to land cover classification have trended toward deep learning in recent years, due to their success in working with large, complex datasets, as well as providing an end-to-end method that encompasses both segmentation and classification. As the volume of high-resolution remote sensing data continues to grow at a remarkable pace, I believe deep learning will be central to improving estuarine classification accuracies while also streamlining the classification process. Both of these improvements will be key, as the library of valuable satellite and aerial imagery collected by NASA and NOAA expands even further.
I also hope the analyses conducted within this research will inform more application-driven research within the field. Shoreline classification results can be used to assess broader estuarine impacts such as shoreline change, land cover change, new setback regulations and vulnerability assessments. As storm-induced erosion continues to pose a major threat to the NRE, I hope to see these applications explored in more in-depth ways, to improve future shoreline management and mitigate hazards.
I look forward to seeing how the field of remote sensing classification improves over the next decade and how results can continue to aid estuarine shoreline communities, as they consider the best actions for recovery from and adaptation to storm-induced erosion.
☽